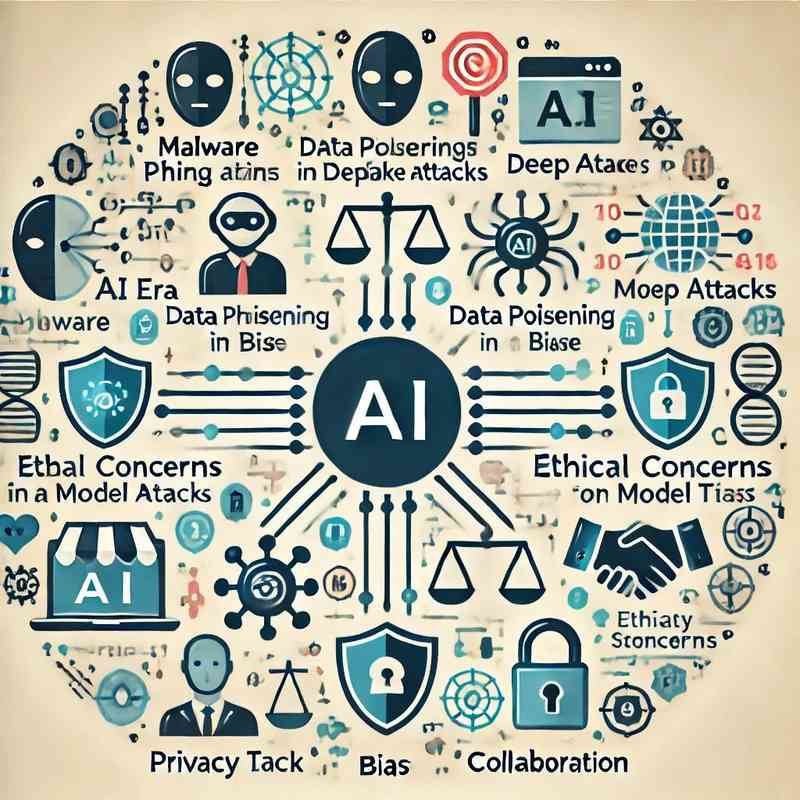
Introduction
The rise of artificial intelligence (AI) is transforming industries worldwide, from healthcare and finance to transportation and entertainment. Yet, while AI offers unprecedented capabilities, it also introduces complex cybersecurity challenges. As AI becomes integral to critical infrastructure, malicious actors can exploit vulnerabilities in AI systems, while ethical concerns around data privacy and transparency add further complexity. This comprehensive overview examines the multifaceted cybersecurity risks unique to the AI era, including real-world case studies, future implications, and strategies to mitigate these challenges.The Evolution of Cybersecurity in the AI Era
The field of cybersecurity has evolved significantly since its inception, with AI playing an increasingly pivotal role. Early cybersecurity measures primarily focused on firewalls, antivirus software, and simple encryption. However, today’s AI-driven systems demand a far more sophisticated approach. Here’s how AI has influenced cybersecurity:
- Automation and Speed: Traditional cybersecurity methods relied on manual intervention, but AI automates threat detection, enabling faster responses.
- Adaptability: AI-driven attacks evolve over time, making static defenses like firewalls insufficient.
- Complexity: Modern AI models, with millions of parameters, introduce complex attack surfaces that traditional cybersecurity was never designed to handle.
Key Cybersecurity Challenges in the AI Era
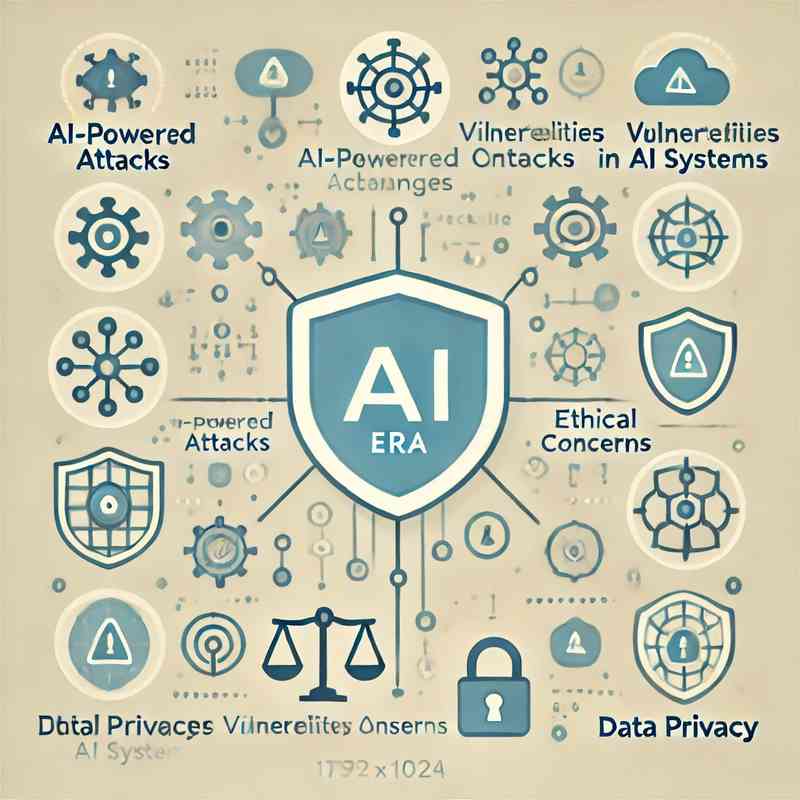
1. AI-Powered Cyberattacks
AI has made cyberattacks more sophisticated and targeted, enabling attackers to exploit system weaknesses at unprecedented levels.
- Sophisticated Malware: AI can generate highly adaptive malware that evolves in response to detection methods, avoiding common antivirus and security protocols.
- Example: IBM’s DeepLocker is a prime example of AI-driven malware. DeepLocker uses deep learning to camouflage itself within benign applications, remaining dormant until it identifies its target. Such adaptive malware could bypass traditional defenses.
- Automated Phishing Attacks: AI algorithms generate highly personalized phishing emails by analyzing social media profiles and behavioral data, making them more convincing and harder to detect.
- Case Study: A 2019 AI-powered phishing campaign targeted thousands of employees from a global organization, adapting messages based on each recipient’s position and social profiles, leading to a 60% click-through rate.
- Deepfakes and Social Engineering: AI enables the creation of realistic deepfakes, often used to impersonate executives or prominent figures, which can result in fraudulent transactions or reputational damage.
- Case Study: In 2020, a CEO in Europe was tricked into transferring $240,000 to criminals who used AI to mimic the voice of his boss, resulting in significant financial loss.
2. Vulnerabilities in AI Systems
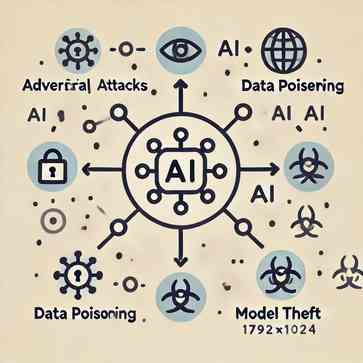
AI models, while powerful, have unique vulnerabilities that attackers can exploit, often through methods such as adversarial attacks and data poisoning.
- Adversarial Attacks: Attackers introduce slight changes to data inputs to manipulate an AI model’s output. These changes may be imperceptible to humans but can lead to misclassification or incorrect decisions by the AI.
- Example: In 2019, researchers showed that placing a small sticker on a stop sign could cause an autonomous vehicle’s AI system to interpret it as a speed limit sign, demonstrating how subtle changes can affect AI reliability.
- Data Poisoning: In a data poisoning attack, attackers inject malicious data into training datasets, compromising the AI model’s accuracy and reliability.
- Example: Google has highlighted this risk in large AI models used for image recognition. By introducing biased or incorrect data, attackers can manipulate outputs, leading to incorrect or dangerous outcomes in areas like facial recognition.
- Model Theft: AI models are often proprietary, containing intellectual property that is valuable to organizations. Model theft involves attackers stealing these models, compromising privacy and security.
- Implications: Stolen models can be reverse-engineered and used for malicious purposes or to create unauthorized copies, diminishing a company’s competitive edge.
3. Ethical Concerns and Bias
Ethical considerations are becoming increasingly prominent in AI cybersecurity, particularly as AI is used for decision-making in sensitive areas like hiring, healthcare, and criminal justice.
- Algorithmic Bias: AI systems can reinforce biases present in training data, leading to unfair treatment of certain groups or demographics.
- Example: Studies have shown that facial recognition algorithms are often less accurate for people of color, leading to concerns about AI use in law enforcement and public surveillance.
- Privacy Violations: AI-powered surveillance and data analysis tools raise concerns about privacy and the potential for misuse of personal data.
- Example: The widespread use of facial recognition in public spaces, such as in China, has led to debates over privacy rights versus security needs.
- Lack of Transparency: AI’s complexity makes it difficult to explain how certain decisions are made, leading to what’s known as the “black box” problem.
- Implication: In fields like healthcare, this lack of transparency can hinder trust in AI-driven diagnoses and treatments.
Mitigating Cybersecurity Risks in the AI Era
Effectively addressing AI-related cybersecurity risks requires a multifaceted approach that includes strong development practices, advanced security measures, and collaboration across industries.
1. Robust AI Development Practices
AI developers must build secure models to withstand cyberattacks and protect data integrity.
- Rigorous Testing and Validation: Comprehensive testing of AI systems helps identify and address vulnerabilities before they are exploited.
- Adversarial Training: Training AI models with adversarial inputs improves their resilience against attacks.
- Continuous Monitoring: Ongoing monitoring ensures AI models function as intended and that anomalies are detected in real time.
2. Enhanced Security Measures
Organizations must implement stringent cybersecurity measures to protect AI systems and data.
- Network Security Protocols: Strong network security measures prevent unauthorized access to AI systems.
- Advanced Threat Detection: AI-powered tools can monitor and detect unusual activities, enhancing threat response.
- Example: Cisco’s AI-driven cybersecurity tools automate responses to low-level threats, allowing teams to focus on more complex issues.
- Regular Security Audits: Frequent assessments help organizations identify and address vulnerabilities.
3. AI-Powered Security Solutions
Using AI-driven security solutions can help organizations combat AI-related threats.
- Automated Threat Intelligence: AI tools that analyze threat data can identify emerging risks and adapt to evolving attack patterns.
- Example: Darktrace uses AI to monitor networks and detect potential threats, providing real-time alerts.
- Behavioral Analytics: AI analyzes user behavior patterns to detect insider threats and prevent unauthorized access.
4. Collaboration and Information Sharing
Collaboration across industries and borders is essential to address global cyber threats effectively.
- Threat Intelligence Sharing: Organizations can benefit from sharing threat intelligence to better understand emerging threats and trends.
- Resource: EC-Council University provides insights on cybersecurity threats and encourages collaboration to tackle AI-driven threats.
- International Cooperation: Global collaboration ensures that cybersecurity solutions remain adaptable across regulatory environments.
Real-World Case Studies
Financial Sector: JPMorgan Chase
JPMorgan Chase leverages AI to detect fraudulent transactions, enhancing real-time monitoring and customer data security. By analyzing transaction patterns with AI, the bank can identify suspicious activities and prevent financial loss.
Healthcare Sector: The Mayo Clinic
The Mayo Clinic uses AI-driven cybersecurity tools to safeguard patient data and prevent unauthorized access. With AI, the clinic can monitor access patterns and detect breaches, ensuring patient privacy and data security.
Government and National Security: Department of Defense
The United States Department of Defense (DoD) utilizes AI to monitor and protect critical infrastructure. AI-driven tools help the DoD detect potential cyber threats and enhance national security by monitoring large-scale networks.
The Future of Cybersecurity in the AI Era
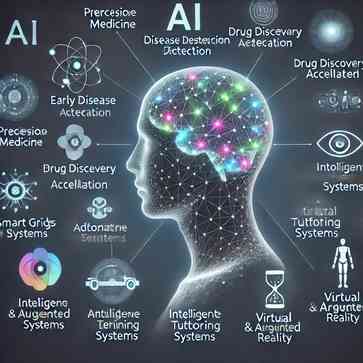
As AI technology continues to evolve, so too will cybersecurity strategies:
- AI-Powered Predictive Analytics: Predictive analytics will help organizations identify and prevent threats before they occur, reducing the likelihood of large-scale breaches.
- Blockchain and AI Integration: Combining AI with blockchain technology will create a more transparent and secure data environment, minimizing risks.
- Global Regulations and Standards: Countries are increasingly adopting AI-specific regulations, similar to GDPR, to protect citizens’ data and privacy.
Title: Cybersecurity Challenges in the AI Era: A Comprehensive Overview
Meta Description: Explore the cybersecurity challenges in the AI era, from AI-powered attacks to privacy concerns, and learn effective mitigation strategies for a secure digital future.
Introduction
The rapid advancement of artificial intelligence (AI) has revolutionized industries, bringing transformative capabilities in data processing, automation, and decision-making. However, with these benefits come new cybersecurity challenges. AI’s integration into critical infrastructure introduces vulnerabilities that malicious actors can exploit, and the increasing sophistication of AI systems has made traditional security measures insufficient. In this post, we’ll explore the cybersecurity risks unique to the AI era, from AI-powered attacks to ethical concerns, and outline strategies for managing these threats.
Key Cybersecurity Challenges in the AI Era
1. AI-Powered Attacks
AI technologies can enhance the capabilities of cyber attackers by making their attacks more sophisticated, adaptive, and difficult to detect.
- Sophisticated Malware: AI can generate malware that adapts in real time, evading traditional detection methods.
- Example: IBM’s DeepLocker AI-powered malware is a proof of concept that remains dormant until it identifies its target, making it hard to detect.
- Automated Phishing Attacks: AI can automate the generation of personalized phishing emails that closely mimic legitimate communication, increasing the likelihood of success.
- Deepfakes and Social Engineering: AI-generated deepfake technology creates convincing fake videos and audio clips, leading to cases of fraud and identity theft.
- Case Study: In 2020, a European energy executive was tricked by a deepfake audio call into transferring $240,000 to cybercriminals who impersonated the voice of his boss.
2. Vulnerabilities in AI Systems
AI models themselves are vulnerable to attacks, which can compromise their effectiveness and reliability.
- Adversarial Attacks: Attackers manipulate AI models by introducing subtle changes to data inputs, leading to incorrect or harmful outcomes.
- Example: In autonomous vehicles, adversarial attacks can manipulate a car’s AI to misinterpret road signs, endangering passengers.
- Data Poisoning: Corrupting training data can compromise the accuracy of AI models, leading to inaccurate or biased outcomes.
- Model Theft: Intellectual property theft of AI models allows unauthorized parties to use or modify proprietary algorithms.
- Example: Attackers can steal models for applications like facial recognition, which could lead to unauthorized surveillance.
3. Ethical Concerns and Bias
AI systems often reflect the biases inherent in the data used to train them, which can perpetuate discriminatory outcomes and privacy violations.
- Algorithmic Bias: AI systems can amplify biases present in training data, leading to unfair or discriminatory decisions.
- Example: In hiring algorithms, biased training data may lead to unfair discrimination against specific demographics, reinforcing existing societal inequalities.
- Privacy Violations: AI-powered surveillance systems can infringe on personal privacy, leading to ethical concerns.
- External Reading: KPMG Netherlands highlights how AI in surveillance raises questions about privacy and civil liberties.
- Lack of Transparency: The complexity of AI algorithms makes it difficult to trace decisions, reducing accountability.
- Implication: As AI-driven decision-making becomes more common, transparency will be essential for fostering public trust.
Mitigating Cybersecurity Risks in the AI Era
Effectively addressing AI-related cybersecurity risks requires a multifaceted approach that includes strong development practices, advanced security measures, and collaboration across industries.
1. Robust AI Development Practices
AI developers must build secure models to withstand cyberattacks and protect data integrity.
- Rigorous Testing and Validation: Comprehensive testing of AI systems helps identify and address vulnerabilities before they are exploited.
- Adversarial Training: Training AI models with adversarial inputs improves their resilience against attacks.
- Continuous Monitoring: Ongoing monitoring ensures AI models function as intended and that anomalies are detected in real time.
2. Enhanced Security Measures
Organizations must implement stringent cybersecurity measures to protect AI systems and data.
- Network Security Protocols: Strong network security measures prevent unauthorized access to AI systems.
- Advanced Threat Detection: AI-powered tools can monitor and detect unusual activities, enhancing threat response.
- Example: Cisco’s AI-driven cybersecurity tools automate responses to low-level threats, allowing teams to focus on more complex issues.
- Regular Security Audits: Frequent assessments help organizations identify and address vulnerabilities.
3. AI-Powered Security Solutions
Using AI-driven security solutions can help organizations combat AI-related threats.
- Automated Threat Intelligence: AI tools that analyze threat data can identify emerging risks and adapt to evolving attack patterns.
- Example: Darktrace uses AI to monitor networks and detect potential threats, providing real-time alerts.
- Behavioral Analytics: AI analyzes user behavior patterns to detect insider threats and prevent unauthorized access.
4. Collaboration and Information Sharing
Collaboration across industries and borders is essential to address global cyber threats effectively.
- Threat Intelligence Sharing: Organizations can benefit from sharing threat intelligence to better understand emerging threats and trends.
- Resource: EC-Council University provides insights on cybersecurity threats and encourages collaboration to tackle AI-driven threats.
- International Cooperation: Global collaboration ensures that cybersecurity solutions remain adaptable across regulatory environments.
Real-World Case Studies
Financial Sector: JPMorgan Chase
JPMorgan Chase leverages AI to detect fraudulent transactions, enhancing real-time monitoring and customer data security. By analyzing transaction patterns with AI, the bank can identify suspicious activities and prevent financial loss.
Healthcare Sector: The Mayo Clinic
The Mayo Clinic uses AI-driven cybersecurity tools to safeguard patient data and prevent unauthorized access. With AI, the clinic can monitor access patterns and detect breaches, ensuring patient privacy and data security.
Government and National Security: Department of Defense
The United States Department of Defense (DoD) utilizes AI to monitor and protect critical infrastructure. AI-driven tools help the DoD detect potential cyber threats and enhance national security by monitoring large-scale networks.
The Future of Cybersecurity in the AI Era
As AI technology continues to evolve, so too will cybersecurity strategies:
- AI-Powered Predictive Analytics: Predictive analytics will help organizations identify and prevent threats before they occur, reducing the likelihood of large-scale breaches.
- Blockchain and AI Integration: Combining AI with blockchain technology will create a more transparent and secure data environment, minimizing risks.
- Global Regulations and Standards: Countries are increasingly adopting AI-specific regulations, similar to GDPR, to protect citizens’ data and privacy.
Conclusion
The AI era has brought significant benefits, but it has also introduced complex cybersecurity challenges. From AI-powered attacks and adversarial vulnerabilities to ethical concerns, securing AI systems requires a multifaceted approach that prioritizes robust development practices, collaboration, and advanced security measures. As we navigate the evolving cybersecurity landscape, organizations and individuals must work together to leverage AI’s potential while ensuring a secure and ethical digital future.
FAQ
Q1: What are the primary cybersecurity challenges in the AI era?
A1: Key challenges include AI-driven cyberattacks, vulnerabilities in AI models, data privacy concerns, and ethical issues related to bias and transparency.
Q2: How can AI improve cybersecurity?
A2: AI enhances cybersecurity by detecting threats faster, automating responses, and identifying patterns in large datasets that indicate potential breaches.
Q3: Which industries are most affected by AI cybersecurity risks?
A3: High-risk industries include finance, healthcare, and government, where sensitive data is frequently processed and targeted by cybercriminals.
Q4: What is an adversarial attack on AI?
A4: An adversarial attack involves manipulating AI models by introducing subtle changes to data inputs, causing the AI to produce incorrect or harmful outputs.
Q5: How can organizations mitigate AI-related cybersecurity risks?
A5: Organizations can implement strong development practices, use AI-powered security solutions, conduct regular audits, and collaborate to share threat intelligence.